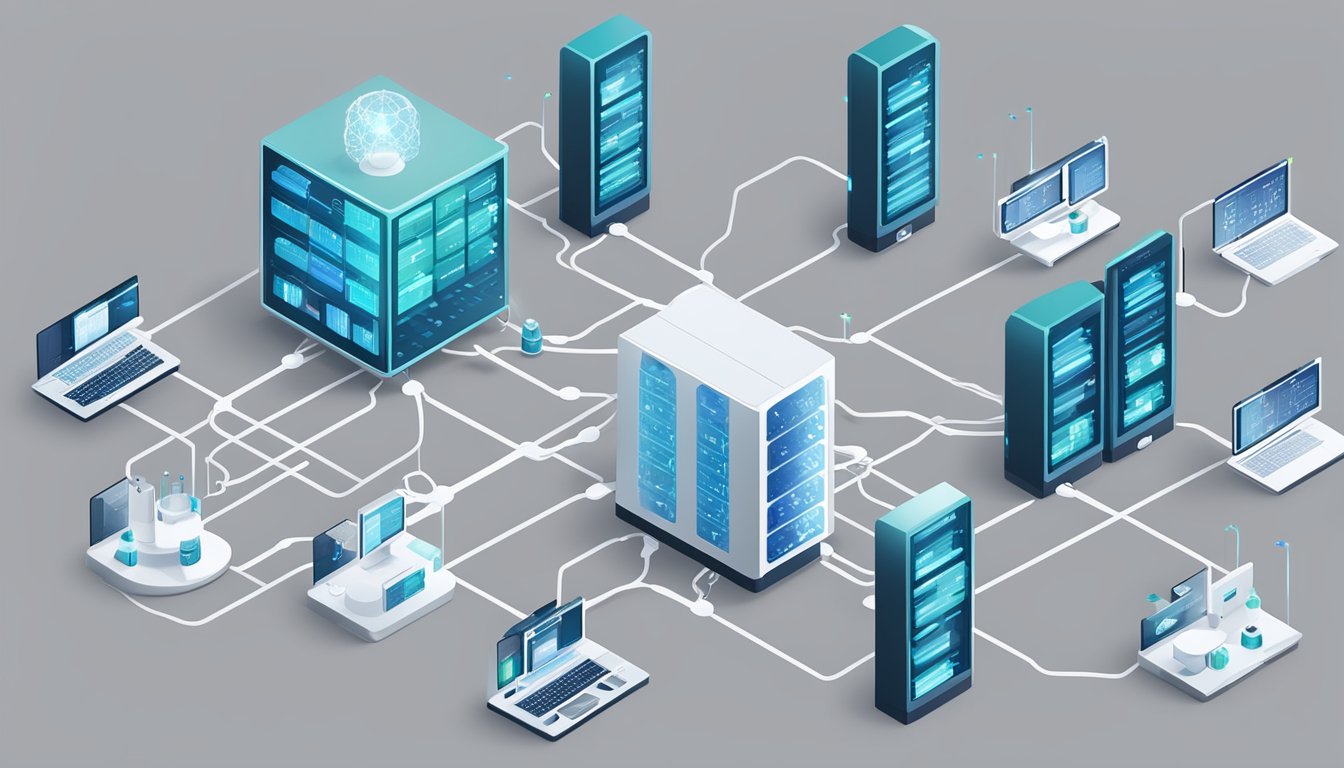
Big Data for Health: Transforming Patient Care Through Advanced Analytics
Big data is revolutionising how healthcare providers deliver services and manage patient care. By integrating vast amounts of information from various sources, healthcare systems can enhance decision-making and improve patient outcomes. The use of big data analytics allows doctors and medical institutions to predict trends in patient health and refine treatment strategies.
Advanced analytics, such as machine learning and artificial intelligence, are crucial for processing and interpreting complex data sets. Hospitals and clinics are now harnessing these technologies to streamline patient care workflows and optimise resources. The continuing development and application of these technologies hold promise for further advancements in healthcare delivery.
In addition to improving current practices, big data is paving the way for innovations in preventive care. By analysing patient data trends, healthcare providers can identify potential health risks before they become critical issues. The increased efficiency and insights offered by big data stand to transform patient care, making it more proactive and personalised.
Key Takeaways
- Big data enhances healthcare decision-making.
- Advanced analytics optimise patient care.
- Data trends aid in preventive healthcare.
Concepts and Fundamentals of Big Data
Big data in healthcare uses advanced analytics to improve patient outcomes and operational efficiency. It involves managing a vast amount of information that must be processed quickly and accurately. Key concepts include the evolution of data storage and analysis, as well as understanding the five essential characteristics of big data.
Evolution of Data in Healthcare
The journey of data in healthcare has significantly evolved over the years. Initially, patient records were paper-based, which posed challenges in data sharing and storage. With the advent of electronic health records (EHRs), the digitisation of patient data became possible. This shift improved accessibility and allowed healthcare professionals to track patient history more efficiently.
The move towards digital storage also paved the way for innovations in medical research and treatment. Now, healthcare providers utilise various data sources, such as sensors and wearable devices, to gather real-time patient information. These technologies have enabled a more personalised approach to patient care, where treatments can be tailored to individual needs based on the data collected.
The Five Vs: Volume, Velocity, Variety, Veracity, and Value
Big data is characterised by five main attributes, often referred to as the “Five Vs”. Volume refers to the extensive amount of data generated daily. In healthcare, this includes everything from patient records to imaging files. Velocityrelates to the speed at which data is processed and analysed to ensure timely decision-making and care delivery.
Variety encompasses the different data types, including structured data like databases and unstructured data such as social media inputs. Veracity addresses the quality and trustworthiness of the data, crucial for accurate diagnoses and treatment plans. Lastly, Value indicates the potential benefits that can be derived, transforming raw data into actionable insights that enhance patient care and healthcare operations.
Big Data Sources in Healthcare
Big data in healthcare comes from numerous sources that each provide valuable information for improving patient care. Key data sources include Electronic Health Records, genomic data, wearable technology, medical imaging, and pharmacy records. Each of these sources presents distinct opportunities and challenges for enhancing healthcare delivery.
Electronic Health Records (EHRs)
Electronic Health Records are digital versions of patients’ paper charts. They contain comprehensive patient data, including medical history, diagnoses, medications, and treatment plans. EHRs make it easier for healthcare providers to access and share patient information, leading to improved coordination and quality of care.
The integration of EHRs into healthcare facilities has enabled the collection of large volumes of patient data. This data is useful for tracking patient outcomes and identifying trends. It also supports the implementation of personalised treatment plans that are more effective in managing chronic conditions.
Genomic Data
Genomic data provides insights into the genetic makeup of individuals. This information helps in predicting disease risk, understanding disease progression, and tailoring treatments to individual genetic profiles. Advances in genomic sequencing technologies have made it possible to collect and analyse vast amounts of genetic data.
This data source is crucial for research in precision medicine. By analysing genomic data, healthcare professionals can develop targeted therapies that are more effective and have fewer side effects. It also supports the discovery of new genetic markers for diseases, leading to early detection and prevention strategies.
Wearable Technology and IoT
Wearable technology and the Internet of Things (IoT) generate continuous streams of health data. Devices such as fitness trackers and smartwatches monitor vital signs, physical activity, and sleep patterns. This real-time data offers valuable insights into a patient’s daily health habits.
The data collected from these devices can be used to detect health issues early and personalise care plans. Healthcare providers can monitor patients remotely, reducing the need for in-person visits. This approach enhances patient engagement and allows for timely interventions when critical changes in health are detected.
Medical Imaging
Medical imaging produces high-resolution images of the inside of the body, which are essential for diagnosis and treatment planning. Technologies like X-rays, MRIs, and CT scans create detailed images that help in detecting illnesses at early stages. The data from medical imaging is massive and requires advanced analytics to interpret effectively.
Machine learning algorithms are increasingly used to analyse medical images, providing more accurate and faster interpretations. This enhances the ability to detect abnormalities and improves diagnostic accuracy. Medical imaging data also helps in tracking disease progression and evaluating the effectiveness of treatments.
Pharmacy Records
Pharmacy records contain detailed information about medications prescribed and dispensed to patients. These records help in tracking medication adherence and identifying patterns in drug usage. Analysing pharmacy data offers insights into prescription trends and helps in managing drug inventories efficiently.
Pharmacy data can be used to monitor drug interactions and side effects, enhancing medication safety. It also supports the identification of issues like over-prescription or potential misuse of medications, allowing healthcare providers to address these concerns effectively.
Data Analytics in Healthcare
Data analytics in healthcare is transforming the industry by providing better insights into patient care, predicting health outcomes, and helping in decision-making. Understanding the specific types of analytics is crucial to see how they contribute to achieving these goals.
Descriptive Analytics
Descriptive analytics in healthcare focuses on summarising past data to identify patterns and trends. It provides a clear picture of what has happened in a healthcare setting. For instance, hospitals use descriptive analytics to track patient admission rates, treatment outcomes, and resource usage.
Clinical dashboards are often employed to visualise this data, making it easier for healthcare professionals to interpret. By analysing historical health records, providers can understand disease prevalence and the efficiency of treatments offered. Hospitals can improve patient satisfaction and operational efficiency through these analyses.
Predictive Analytics
Predictive analytics takes data a step further by using statistical models to forecast future events. It is particularly valuable in anticipating patient outcomes and risks. For example, predictive models can identify patients at high risk for readmission, allowing for preemptive interventions.
Machine learning algorithms are frequently used to enhance the accuracy of predictions by analysing large datasets. Healthcare providers can thereby better allocate resources and personalise patient care. Predictive analytics also plays a key role in population health management, as it helps identify emerging health trends.
Prescriptive Analytics
Prescriptive analytics suggests actions to optimise outcomes, not just predicting them. It builds on the insights of descriptive and predictive analyses to recommend specific actions. For instance, it can suggest the best treatment plans for individual patients based on their medical history and current data.
By integrating data from multiple sources, including electronic health records and wearable devices, prescriptive analytics helps in making informed clinical decisions. Optimisation and simulation tools are often used to evaluate different treatment scenarios, guiding healthcare professionals towards the most effective strategies. This approach ultimately supports better patient outcomes and cost management strategies.
Big Data Technologies
Big Data Technologies are crucial in health care, enabling the storage, processing, and analysis of vast datasets. Key technologies include data warehousing for centralised storage, Hadoop for distributed processing, NoSQL databases for flexible data models, and cloud computing for scalable services.
Data Warehousing
Data warehousing involves the collection and management of large amounts of data from different sources. These systems store data in a structured way, making it easier for healthcare providers to access and analyse information. By consolidating data into a central repository, health organisations can improve patient management and optimise decision-making processes. The integration of various data types, such as patient records and treatment histories, allows for comprehensive insights into patient care. Data warehouses also support complex queries and reporting, facilitating advanced analytics and fostering an environment of informed healthcare practices.
Hadoop and MapReduce Frameworks
Hadoop is an open-source software framework that enables the processing of large datasets across distributed computing environments. Within healthcare, Hadoop and MapReduce frameworks assist in analysing complex data systems, such as genomic datasets or electronic health records. By distributing the computational workload across multiple nodes, these frameworks enhance efficiency and speed. Hadoop’s ability to store and process large amounts of data at a lower cost is particularly beneficial for institutions with budget constraints. MapReduce allows for efficient data processing and analysis tasks by breaking them down into smaller, manageable segments. This scalability and cost-effectiveness make Hadoop a valuable tool in managing big data in healthcare.
NoSQL Databases
NoSQL databases provide a flexible approach to storing unstructured and semi-structured data. Unlike traditional relational databases, NoSQL can handle large volumes of diverse data, such as text, images, and social media inputs. In the healthcare sector, this flexibility is crucial for managing data that does not fit into standard database schemas. Hospitals and clinics can benefit from NoSQL’s ability to quickly adapt to evolving data requirements, offering a significant degree of customisation. The ability to scale horizontally ensures that healthcare providers can meet increasing data demands without sacrificing performance. NoSQL databases are instrumental in supporting real-time data access and analysis.
Cloud Computing Services
Cloud computing offers scalable and flexible resources for handling and processing large amounts of data in healthcare. By leveraging cloud services, healthcare organisations can store extensive datasets without investing in physical infrastructure. This approach allows for remote data access, improving collaboration among healthcare providers and researchers. Cloud computing also supports powerful analytics tools that can process big data efficiently, leading to faster and more accurate decision-making. Security and compliance are key considerations, and many cloud service providers offer robust measures to protect patient data. The ability to scale resources as needed makes cloud computing an attractive option for dynamic healthcare environments.
Implementation Challenges
Implementing big data analytics in healthcare presents several hurdles that must be addressed. These challenges include issues with data privacy and security, difficulties in data integration and interoperability, ethical dilemmas, and the need for robust technical infrastructure.
Data Privacy and Security
Protecting patient information is a primary concern. Big data involves gathering vast amounts of data from various sources. This can expose sensitive information to potential breaches. Securing data is vital to maintaining trust in healthcare systems. Encryption and access controls are common strategies to safeguard data.
Regulations like the GDPR in Europe mandate strict data protection measures. Compliance with such regulations requires ongoing monitoring and updates to security protocols. Breaches in data privacy can not only lead to legal consequences but also damage reputations.
Data Integration and Interoperability
Integrating data from different systems is complex. Healthcare systems often use varied technologies and standards. These differences make combining data challenging. Ensuring interoperability is crucial for a unified approach to patient care.
Frameworks enabling data exchange between platforms are necessary. Standardised protocols, like HL7 and FHIR, aim to simplify this process. They help in reducing technical barriers. Despite these efforts, achieving seamless integration remains a continuous challenge due to the diversity of healthcare IT systems.
Ethical Considerations
The use of big data in healthcare raises ethical questions. Concerns about consent and patient autonomy are prominent. Patients should be aware of how their data is used and have control over it. Transparent data practices help in maintaining ethical standards.
Balancing patient privacy with the benefits of big data is crucial. Ethical frameworks guide how data should be collected and used responsibly. Implementation of such frameworks requires ongoing dialogue among stakeholders. This ensures that ethical considerations are always at the forefront.
Technical Infrastructure
Robust technical infrastructure is essential for handling large datasets. Healthcare organisations must invest in advanced technologies to store and process big data efficiently. This requires significant resource allocation and expertise.
Cloud computing and scalable databases are often used to manage data. However, maintaining these systems can be costly and complex. Ensuring reliability and high performance is critical to the successful implementation of big data analytics. A lack of infrastructure can impede data-driven projects and limit potential benefits.
Applications of Big Data in Healthcare
Big Data is transforming healthcare by improving patient outcomes, enhancing operational efficiencies, and driving research innovations. These applications are vital in tailoring treatments, managing population health, supporting clinical decisions, and optimising healthcare operations.
Personalised Medicine
Personalised medicine leverages Big Data to tailor treatments to individual patients. By analysing genetic, lifestyle, and environmental information, healthcare providers can predict an individual’s susceptibility to certain diseases, choose the most effective treatments, and avoid adverse drug reactions. Machine learning algorithms are employed to interpret vast amounts of patient data, enabling more precise and targeted interventions. This approach is creating a shift from one-size-fits-all treatments to customised care plans, making healthcare more effective and personalised.
Population Health Management
Population health management involves using Big Data to monitor and improve the health outcomes of groups. By aggregating data from various sources such as electronic health records (EHRs), social determinants of health, and wearable technology, trends and patterns in health issues are identified. This data-driven approach helps in planning intervention strategies, allocating resources efficiently, and predicting outbreaks. It also supports the development of preventative measures, ultimately reducing healthcare costs and improving public health.
Clinical Decision Support Systems
Clinical decision support systems (CDSS) enhance clinical decision-making through Big Data analytics. These systems provide medical professionals with evidence-based recommendations by analysing patient data alongside current research evidence. By offering insights on diagnosis, treatment options, and potential drug interactions, CDSS improves the quality of patient care. The integration of such systems into EHRs helps reduce errors, standardise care, and streamline workflow, enabling clinicians to make informed and timely decisions.
Operational Efficiency Improvements
Operational efficiency in healthcare facilities is significantly enhanced through the use of Big Data. By analysing data on patient flow, staff performance, and resource utilisation, hospitals can optimise their operations. Predictive analytics helps in forecasting demand for services and managing inventories, leading to cost reductions and improved patient satisfaction. Furthermore, scheduling and staffing can be greatly improved, ensuring that healthcare providers effectively meet patient needs without overworking their staff.
Research and Development
Big Data plays a critical role in healthcare research and development. It allows researchers to analyse large datasets to discover new treatments and understand complex diseases better. In the pharmaceutical industry, Big Data accelerates drug discovery by identifying potential molecular targets and predicting drug efficacy. Collaboration between healthcare providers and research institutions enables the sharing of real-world data, fostering innovation and speeding up the development of new medical solutions.
Impact of Big Data on Healthcare Outcomes
Big data is reshaping healthcare by enhancing the quality of care, reducing costs, and boosting patient engagement. These changes are driven by advanced data analytics, which provide deeper insights into patient management and treatment strategies.
Quality of Care Enhancement
Big data analytics significantly improves the quality of healthcare by allowing providers to personalise treatment plans. With access to vast amounts of data, clinicians can tailor interventions based on specific patient needs. This enables more accurate diagnoses and better management of chronic diseases. Predictive analytics plays a key role in foreseeing potential health risks and taking preventive measures. Advanced tools are used to analyse patient histories, revealing patterns that might not be immediately visible. By identifying at-risk patients early, healthcare providers can mitigate complications and improve outcomes. Real-time data monitoring also aids in immediate decision-making, ensuring timely interventions and enhancing overall care delivery.
Cost Reduction Strategies
Implementing big data solutions can lead healthcare systems to operate more efficiently, ultimately driving down costs. Data analytics help identify unnecessary tests and procedures, paving the way for more streamlined care. By focusing resources on the most effective treatments, hospitals can optimise budgets and reduce waste. Big data also contributes to reducing hospital readmission rates by monitoring patient progress post-discharge. Analysing patient data can highlight trends in resource use, suggesting improvements in operational processes. For instance, predictive analytics can anticipate staffing needs, leading to better resource allocation. These efficiencies not only save money but also improve service delivery by ensuring that resources are available where they are needed most.
Patient Engagement and Satisfaction
Big data enhances patient engagement by giving individuals greater access to their health data. With the ability to track their medical history and receive tailored health advice, patients become more active participants in their care. This increased involvement often leads to higher satisfaction levels as patients feel more in control of their health journey. Personal health apps and wearables collect data that can be shared with clinicians, fostering collaborative care relationships. In turn, healthcare providers can offer personalised recommendations, further enhancing patient experience. As communication between patients and providers becomes more transparent through data sharing, trust and satisfaction are strengthened. This proactive approach encourages patients to remain engaged with their healthcare plans, resulting in better adherence to treatments and improved outcomes.
Case Studies and Success Stories
Big data analytics is transforming healthcare, providing real-time health monitoring, enhancing predictive capabilities for disease outbreaks, and accelerating drug discovery. These advancements offer significant potential for improving patient outcomes and care efficiency.
Real-time Health Monitoring
Real-time health monitoring has greatly benefited from big data analytics. By collecting continuous data from wearable devices, healthcare providers can track vital signs such as heart rate, blood pressure, and activity levels. This constant stream of information allows for immediate response to any alarming changes in a patient’s condition.
For example, some healthcare organisations use big data to monitor patients with chronic illnesses. By analysing patterns in the data, they can predict potential health issues and intervene early to prevent hospital visits. This proactive approach helps in saving costs and improving patient quality of life.
Predictive Analytics for Disease Outbreaks
Predictive analytics plays a critical role in identifying and managing disease outbreaks. By analysing large datasets from various sources, including social media and health records, researchers can detect early signs of an outbreak and take preventive measures.
The use of big data in predicting Ebola outbreaks showed how effective it can be. By tracking travel data and social patterns, health authorities were able to focus resources more efficiently. This method reduced the spread of the virus and improved response times significantly. Similarly, other diseases can be managed better with timely data-driven insights.
Drug Discovery and Development
Drug discovery has traditionally been a time-consuming and costly process. Big data analytics is helping pharmaceutical companies streamline this by sifting through large volumes of data to identify potential drug candidates quickly. For instance, data from clinical trials, genetic profiles, and scientific literature are analysed to discover new drug possibilities.
A notable success includes advancements in personalised medicine. By understanding genetic variations, researchers can tailor drugs to optimise patient response. This not only speeds up the drug development process but also increases the likelihood of success in clinical trials. As a result, new treatments are reaching patients faster than before and providing more precise therapies.
Future Trends and Predictions
Big data is set to redefine health care with its potential for accurate predictions and improved patient outcomes. Health institutions are increasingly adopting advanced analytics to make their systems smarter and more efficient.
Machine learning will play a central role in processing large datasets. By analysing patterns, health care providers can enhance patient care and reduce errors. Predictive models can foresee epidemics, allowing quick response and preventive measures.
Emerging trends show a growing interest in integrating IoT devices with health care systems. These devices will continuously monitor patient health, offering real-time data for timely interventions. They promise to transform treatment approaches and patient monitoring.
Telemedicine is likely to expand further, relying on data analytics for virtual consultations. By using historical data, care can be tailored to individual needs, ensuring more personalised treatments. This shift is expected to make health care more accessible.
Another promising area is the use of big data in genome sequencing. Analytical techniques can identify genetic markers, assisting in early disease detection and personalised medicine strategies. This trend is set to revolutionise preventive care.
As data privacy regulations tighten, health care organisations must prioritise data security. Innovations in encryption and secure data handling will become crucial to maintaining trust and compliance.